Sumanta Basu is an Assistant Professor in the Department of Statistics and Data Science at Cornell University. Broadly, his research interests are structure learning and the prediction of large systems from data, with a particular emphasis on developing learning algorithms for time series data. Professor Basu also collaborates with biological and social scientists on a wide range of problems, including genomics, large-scale metabolomics, and systemic risk monitoring in financial markets. His research is supported by multiple awards from the National Science Foundation and the National Institutes of Health. At Cornell, Professor Basu teaches “Introductory Statistics” for graduate students outside the Statistics Department and “Computational Statistics” for Statistics Ph.D. students. He also serves as a faculty consultant at Cornell Statistical Consulting Unit, which assists the broader Cornell community with various aspects of analyzing empirical research. Professor Basu received his Ph.D. from the University of Michigan and was a postdoctoral scholar at the University of California, Berkeley, and Lawrence Berkeley National Laboratory. Before he received his Ph.D, Professor Basu was a business analyst, working with large retail companies on the design and data analysis of their promotional campaigns.
Foundations of Predictive ModelingCornell Course
Course Overview
The goal of this course is to introduce you to the fundamental concepts and techniques used in predictive modeling. Throughout this course, you will evaluate the balance between model flexibility and interpretability, examine how to select the best parameters using cross-validation, and practice building models that generalize well to new data. You will also explore techniques for splitting datasets, selecting tuning parameters, and fitting models using loss functions. By the end of the course, you will have a solid understanding of model flexibility, interpretability, and the bias-variance trade-off, equipping you to effectively build and evaluate predictive models.
You are required to have completed the following courses or have equivalent experience before taking this course:
- Nonlinear Regression Models
- Modeling Interactions Between Predictors
Key Course Takeaways
- Explore how models have tuning parameters that control their flexibility
- Explain the flexibility-interpretability and bias-variance trade-offs
- Define overfitting and find out why it is a problem for data scientists
- Choose an optimal model and tuning parameters using cross-validation approaches
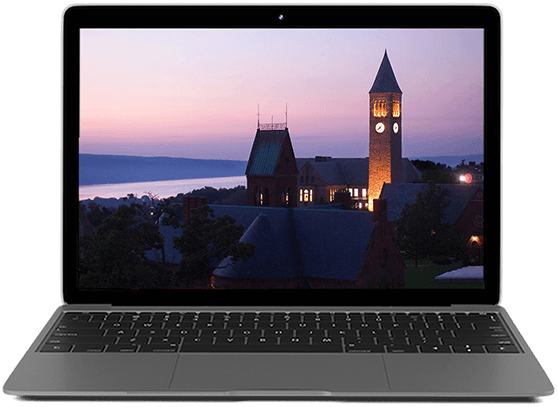
How It Works
Course Author
Who Should Enroll
- Current and aspiring data scientists and analysts
- Business decision makers
- Marketing analysts
- Consultants
- Executives
- Anyone seeking to gain deeper exposure to data science
100% Online
cornell's Top Minds
career